線形回帰診断¶
現実の世界では、応答変数と目的変数の関係が線形であることは稀です。ここでは、statsmodels
の出力を利用して、非線形な関係に 線形回帰
モデルを当てはめる際に発生する可能性のある問題を可視化し、特定します。主な目的は、Jamesらによる著書 An Introduction to Statistical Learning (ISLR) の潜在的な問題 (第3.3.3章) で説明されている可視化を再現することです。
[1]:
import statsmodels
import statsmodels.formula.api as smf
import pandas as pd
単純な重回帰¶
まず、ISLR本の第2章のAdvertisingデータセットを読み込み、線形モデルを当てはめてみましょう。
[2]:
# Load data
data_url = "https://raw.githubusercontent.com/nguyen-toan/ISLR/07fd968ea484b5f6febc7b392a28eb64329a4945/dataset/Advertising.csv"
df = pd.read_csv(data_url).drop('Unnamed: 0', axis=1)
df.head()
[2]:
TV | ラジオ | 新聞 | 売上 | |
---|---|---|---|---|
0 | 230.1 | 37.8 | 69.2 | 22.1 |
1 | 44.5 | 39.3 | 45.1 | 10.4 |
2 | 17.2 | 45.9 | 69.3 | 9.3 |
3 | 151.5 | 41.3 | 58.5 | 18.5 |
4 | 180.8 | 10.8 | 58.4 | 12.9 |
[3]:
# Fitting linear model
res = smf.ols(formula= "Sales ~ TV + Radio + Newspaper", data=df).fit()
res.summary()
[3]:
従属変数 | 売上 | R二乗 | 0.897 |
---|---|---|---|
モデル | OLS | 調整済みR二乗 | 0.896 |
手法 | 最小二乗法 | F統計量 | 570.3 |
日付 | 2024年10月3日(木) | F統計量の確率 | 1.58e-96 |
時間 | 15:45:11 | 対数尤度 | -386.18 |
観測値数 | 200 | AIC | 780.4 |
残差の自由度 | 196 | BIC | 793.6 |
モデルの自由度 | 3 | ||
共分散の種類 | 非ロバスト |
係数 | 標準誤差 | t | P>|t| | [0.025 | 0.975] | |
---|---|---|---|---|---|---|
切片 | 2.9389 | 0.312 | 9.422 | 0.000 | 2.324 | 3.554 |
TV | 0.0458 | 0.001 | 32.809 | 0.000 | 0.043 | 0.049 |
ラジオ | 0.1885 | 0.009 | 21.893 | 0.000 | 0.172 | 0.206 |
新聞 | -0.0010 | 0.006 | -0.177 | 0.860 | -0.013 | 0.011 |
オムニバス | 60.414 | ダービン・ワトソン | 2.084 |
---|---|---|---|
確率(オムニバス) | 0.000 | ジャック・ベラ(JB) | 151.241 |
歪度 | -1.327 | 確率(JB) | 1.44e-33 |
尖度 | 6.332 | 条件数 | 454. |
注釈
[1] 標準誤差は、誤差の共分散行列が正しく指定されていると仮定しています。
診断図/表¶
以下では、診断プロットの生成に使用するベースコードを提示します。
a. residual
b. qq
c. scale location
d. leverage
そして表
a. vif
[4]:
# base code
import numpy as np
import seaborn as sns
from statsmodels.tools.tools import maybe_unwrap_results
from statsmodels.graphics.gofplots import ProbPlot
from statsmodels.stats.outliers_influence import variance_inflation_factor
import matplotlib.pyplot as plt
from typing import Type
style_talk = 'seaborn-talk' #refer to plt.style.available
class LinearRegDiagnostic():
"""
Diagnostic plots to identify potential problems in a linear regression fit.
Mainly,
a. non-linearity of data
b. Correlation of error terms
c. non-constant variance
d. outliers
e. high-leverage points
f. collinearity
Authors:
Prajwal Kafle (p33ajkafle@gmail.com, where 3 = r)
Does not come with any sort of warranty.
Please test the code one your end before using.
Matt Spinelli (m3spinelli@gmail.com, where 3 = r)
(1) Fixed incorrect annotation of the top most extreme residuals in
the Residuals vs Fitted and, especially, the Normal Q-Q plots.
(2) Changed Residuals vs Leverage plot to match closer the y-axis
range shown in the equivalent plot in the R package ggfortify.
(3) Added horizontal line at y=0 in Residuals vs Leverage plot to
match the plots in R package ggfortify and base R.
(4) Added option for placing a vertical guideline on the Residuals
vs Leverage plot using the rule of thumb of h = 2p/n to denote
high leverage (high_leverage_threshold=True).
(5) Added two more ways to compute the Cook's Distance (D) threshold:
* 'baseR': D > 1 and D > 0.5 (default)
* 'convention': D > 4/n
* 'dof': D > 4 / (n - k - 1)
(6) Fixed class name to conform to Pascal casing convention
(7) Fixed Residuals vs Leverage legend to work with loc='best'
"""
def __init__(self,
results: Type[statsmodels.regression.linear_model.RegressionResultsWrapper]) -> None:
"""
For a linear regression model, generates following diagnostic plots:
a. residual
b. qq
c. scale location and
d. leverage
and a table
e. vif
Args:
results (Type[statsmodels.regression.linear_model.RegressionResultsWrapper]):
must be instance of statsmodels.regression.linear_model object
Raises:
TypeError: if instance does not belong to above object
Example:
>>> import numpy as np
>>> import pandas as pd
>>> import statsmodels.formula.api as smf
>>> x = np.linspace(-np.pi, np.pi, 100)
>>> y = 3*x + 8 + np.random.normal(0,1, 100)
>>> df = pd.DataFrame({'x':x, 'y':y})
>>> res = smf.ols(formula= "y ~ x", data=df).fit()
>>> cls = Linear_Reg_Diagnostic(res)
>>> cls(plot_context="seaborn-v0_8-paper")
In case you do not need all plots you can also independently make an individual plot/table
in following ways
>>> cls = Linear_Reg_Diagnostic(res)
>>> cls.residual_plot()
>>> cls.qq_plot()
>>> cls.scale_location_plot()
>>> cls.leverage_plot()
>>> cls.vif_table()
"""
if isinstance(results, statsmodels.regression.linear_model.RegressionResultsWrapper) is False:
raise TypeError("result must be instance of statsmodels.regression.linear_model.RegressionResultsWrapper object")
self.results = maybe_unwrap_results(results)
self.y_true = self.results.model.endog
self.y_predict = self.results.fittedvalues
self.xvar = self.results.model.exog
self.xvar_names = self.results.model.exog_names
self.residual = np.array(self.results.resid)
influence = self.results.get_influence()
self.residual_norm = influence.resid_studentized_internal
self.leverage = influence.hat_matrix_diag
self.cooks_distance = influence.cooks_distance[0]
self.nparams = len(self.results.params)
self.nresids = len(self.residual_norm)
def __call__(self, plot_context='seaborn-v0_8-paper', **kwargs):
# print(plt.style.available)
with plt.style.context(plot_context):
fig, ax = plt.subplots(nrows=2, ncols=2, figsize=(10,10))
self.residual_plot(ax=ax[0,0])
self.qq_plot(ax=ax[0,1])
self.scale_location_plot(ax=ax[1,0])
self.leverage_plot(
ax=ax[1,1],
high_leverage_threshold = kwargs.get('high_leverage_threshold'),
cooks_threshold = kwargs.get('cooks_threshold'))
plt.show()
return self.vif_table(), fig, ax,
def residual_plot(self, ax=None):
"""
Residual vs Fitted Plot
Graphical tool to identify non-linearity.
(Roughly) Horizontal red line is an indicator that the residual has a linear pattern
"""
if ax is None:
fig, ax = plt.subplots()
sns.residplot(
x=self.y_predict,
y=self.residual,
lowess=True,
scatter_kws={'alpha': 0.5},
line_kws={'color': 'red', 'lw': 1, 'alpha': 0.8},
ax=ax)
# annotations
residual_abs = np.abs(self.residual)
abs_resid = np.flip(np.argsort(residual_abs), 0)
abs_resid_top_3 = abs_resid[:3]
for i in abs_resid_top_3:
ax.annotate(
i,
xy=(self.y_predict[i], self.residual[i]),
color='C3')
ax.set_title('Residuals vs Fitted', fontweight="bold")
ax.set_xlabel('Fitted values')
ax.set_ylabel('Residuals')
return ax
def qq_plot(self, ax=None):
"""
Standarized Residual vs Theoretical Quantile plot
Used to visually check if residuals are normally distributed.
Points spread along the diagonal line will suggest so.
"""
if ax is None:
fig, ax = plt.subplots()
QQ = ProbPlot(self.residual_norm)
fig = QQ.qqplot(line='45', alpha=0.5, lw=1, ax=ax)
# annotations
abs_norm_resid = np.flip(np.argsort(np.abs(self.residual_norm)), 0)
abs_norm_resid_top_3 = abs_norm_resid[:3]
for i, x, y in self.__qq_top_resid(QQ.theoretical_quantiles, abs_norm_resid_top_3):
ax.annotate(
i,
xy=(x, y),
ha='right',
color='C3')
ax.set_title('Normal Q-Q', fontweight="bold")
ax.set_xlabel('Theoretical Quantiles')
ax.set_ylabel('Standardized Residuals')
return ax
def scale_location_plot(self, ax=None):
"""
Sqrt(Standarized Residual) vs Fitted values plot
Used to check homoscedasticity of the residuals.
Horizontal line will suggest so.
"""
if ax is None:
fig, ax = plt.subplots()
residual_norm_abs_sqrt = np.sqrt(np.abs(self.residual_norm))
ax.scatter(self.y_predict, residual_norm_abs_sqrt, alpha=0.5);
sns.regplot(
x=self.y_predict,
y=residual_norm_abs_sqrt,
scatter=False, ci=False,
lowess=True,
line_kws={'color': 'red', 'lw': 1, 'alpha': 0.8},
ax=ax)
# annotations
abs_sq_norm_resid = np.flip(np.argsort(residual_norm_abs_sqrt), 0)
abs_sq_norm_resid_top_3 = abs_sq_norm_resid[:3]
for i in abs_sq_norm_resid_top_3:
ax.annotate(
i,
xy=(self.y_predict[i], residual_norm_abs_sqrt[i]),
color='C3')
ax.set_title('Scale-Location', fontweight="bold")
ax.set_xlabel('Fitted values')
ax.set_ylabel(r'$\sqrt{|\mathrm{Standardized\ Residuals}|}$');
return ax
def leverage_plot(self, ax=None, high_leverage_threshold=False, cooks_threshold='baseR'):
"""
Residual vs Leverage plot
Points falling outside Cook's distance curves are considered observation that can sway the fit
aka are influential.
Good to have none outside the curves.
"""
if ax is None:
fig, ax = plt.subplots()
ax.scatter(
self.leverage,
self.residual_norm,
alpha=0.5);
sns.regplot(
x=self.leverage,
y=self.residual_norm,
scatter=False,
ci=False,
lowess=True,
line_kws={'color': 'red', 'lw': 1, 'alpha': 0.8},
ax=ax)
# annotations
leverage_top_3 = np.flip(np.argsort(self.cooks_distance), 0)[:3]
for i in leverage_top_3:
ax.annotate(
i,
xy=(self.leverage[i], self.residual_norm[i]),
color = 'C3')
factors = []
if cooks_threshold == 'baseR' or cooks_threshold is None:
factors = [1, 0.5]
elif cooks_threshold == 'convention':
factors = [4/self.nresids]
elif cooks_threshold == 'dof':
factors = [4/ (self.nresids - self.nparams)]
else:
raise ValueError("threshold_method must be one of the following: 'convention', 'dof', or 'baseR' (default)")
for i, factor in enumerate(factors):
label = "Cook's distance" if i == 0 else None
xtemp, ytemp = self.__cooks_dist_line(factor)
ax.plot(xtemp, ytemp, label=label, lw=1.25, ls='--', color='red')
ax.plot(xtemp, np.negative(ytemp), lw=1.25, ls='--', color='red')
if high_leverage_threshold:
high_leverage = 2 * self.nparams / self.nresids
if max(self.leverage) > high_leverage:
ax.axvline(high_leverage, label='High leverage', ls='-.', color='purple', lw=1)
ax.axhline(0, ls='dotted', color='black', lw=1.25)
ax.set_xlim(0, max(self.leverage)+0.01)
ax.set_ylim(min(self.residual_norm)-0.1, max(self.residual_norm)+0.1)
ax.set_title('Residuals vs Leverage', fontweight="bold")
ax.set_xlabel('Leverage')
ax.set_ylabel('Standardized Residuals')
plt.legend(loc='best')
return ax
def vif_table(self):
"""
VIF table
VIF, the variance inflation factor, is a measure of multicollinearity.
VIF > 5 for a variable indicates that it is highly collinear with the
other input variables.
"""
vif_df = pd.DataFrame()
vif_df["Features"] = self.xvar_names
vif_df["VIF Factor"] = [variance_inflation_factor(self.xvar, i) for i in range(self.xvar.shape[1])]
return (vif_df
.sort_values("VIF Factor")
.round(2))
def __cooks_dist_line(self, factor):
"""
Helper function for plotting Cook's distance curves
"""
p = self.nparams
formula = lambda x: np.sqrt((factor * p * (1 - x)) / x)
x = np.linspace(0.001, max(self.leverage), 50)
y = formula(x)
return x, y
def __qq_top_resid(self, quantiles, top_residual_indices):
"""
Helper generator function yielding the index and coordinates
"""
offset = 0
quant_index = 0
previous_is_negative = None
for resid_index in top_residual_indices:
y = self.residual_norm[resid_index]
is_negative = y < 0
if previous_is_negative == None or previous_is_negative == is_negative:
offset += 1
else:
quant_index -= offset
x = quantiles[quant_index] if is_negative else np.flip(quantiles, 0)[quant_index]
quant_index += 1
previous_is_negative = is_negative
yield resid_index, x, y
を利用して
* fitted model on the Advertising data above and
* the base code provided
これで、診断プロットを1つずつ生成します。
[5]:
cls = LinearRegDiagnostic(res)
A. 残差 vs 適合値
非線形性を特定するためのグラフツールです。
グラフの赤色の(おおよそ)水平線は、残差が線形のパターンを持っていることを示しています。
[6]:
cls.residual_plot();
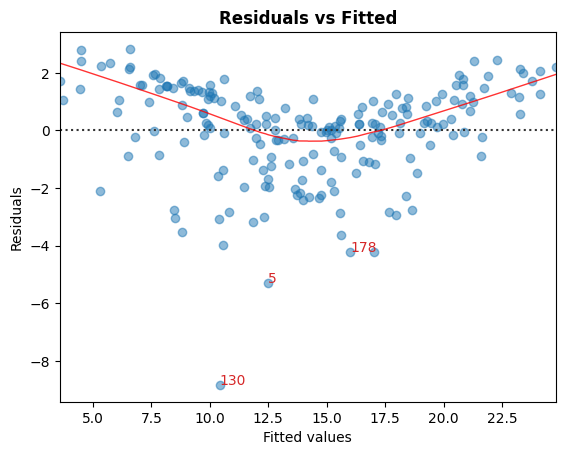
B. 標準化された残差 vs 理論上の分位数
このプロットは、残差が正規分布に従っているかどうかを目視で確認するために使用されます。
対角線に沿って点が広がっていれば、そうである可能性を示唆します。
[7]:
cls.qq_plot();
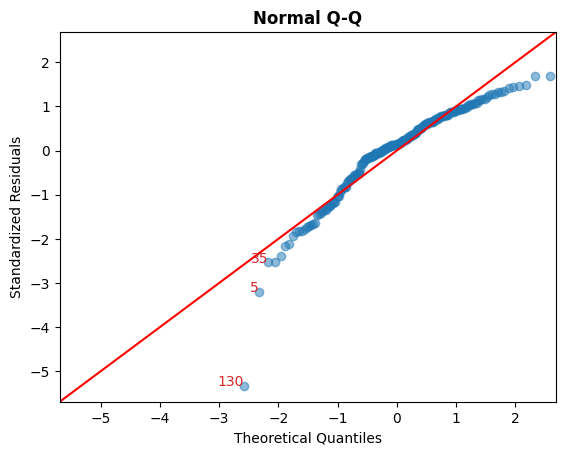
C. sqrt(標準化された残差) vs 適合値
このプロットは、残差の等分散性を確認するために使用されます。
グラフ内のほぼ水平な赤線は、そうであることを示唆します。
[8]:
cls.scale_location_plot();
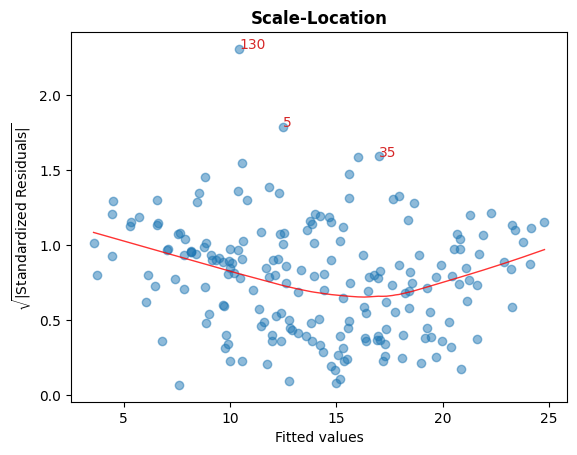
D. 残差 vs てこ比
クックの距離曲線から外れている点は、適合に影響を与える可能性のある観測値、つまり影響力のある観測値と見なされます。
これらの曲線の外側に点がないのが望ましいです。
[9]:
cls.leverage_plot();
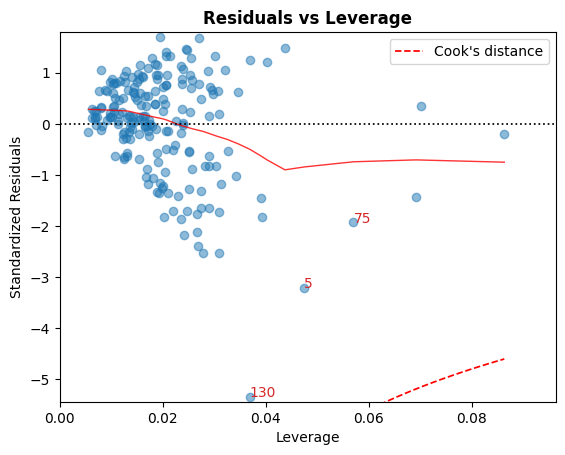
クックの距離曲線は、他の経験則を使用して描画することもできます
経験則 |
しきい値 |
---|---|
|
\[D_i > 1 \mid D_i > 0.5\]
|
|
\[D_i > { 4 \over n}\]
|
|
\[D_i > {4 \over n - k - 1}\]
|
高いてこ比のガイドラインは、慣例: \(h_{ii} > {2p \over n}\) を使用して表示することもできます。
[10]:
cls.leverage_plot(high_leverage_threshold=True, cooks_threshold='dof');
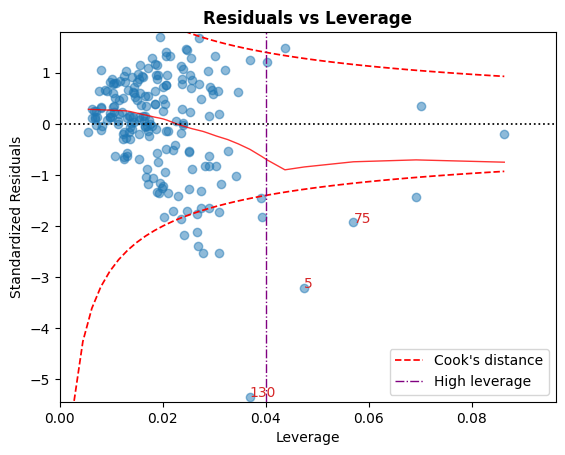
E. VIF
分散拡大係数 (VIF) は、多重共線性の尺度です。
変数のVIF > 5は、その変数が他の入力変数と高度に共線していることを示します。
[11]:
cls.vif_table()
[11]:
特徴 | VIF係数 | |
---|---|---|
1 | TV | 1.00 |
2 | ラジオ | 1.14 |
3 | 新聞 | 1.15 |
0 | 切片 | 6.85 |
[12]:
# Alternatively, all diagnostics can be generated in one go as follows.
# Fig and ax can be used to modify axes or plot properties after the fact.
cls = LinearRegDiagnostic(res)
vif, fig, ax = cls()
print(vif)
#fig.savefig('../../docs/source/_static/images/linear_regression_diagnostics_plots.png')
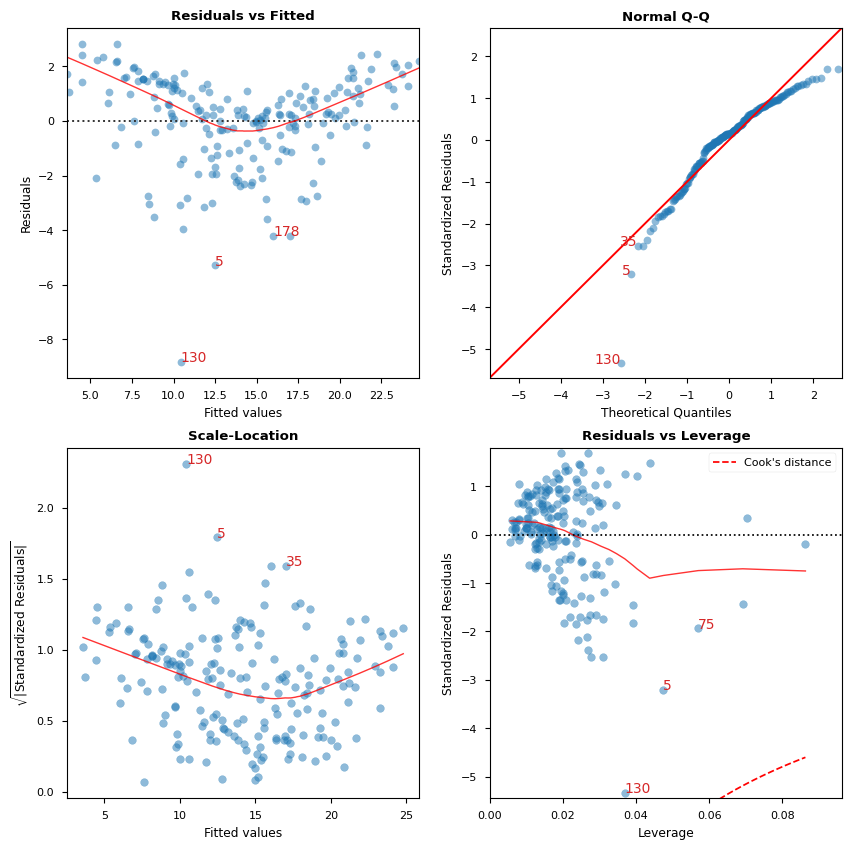
Features VIF Factor
1 TV 1.00
2 Radio 1.14
3 Newspaper 1.15
0 Intercept 6.85
上記のプロットの解釈と注意点に関する詳細な説明については、ISLR本を参照してください。