LOESSを用いた季節・トレンド分解 (STL)¶
このノートブックは、STL
を使用して時系列をトレンド、季節、残差の3つの成分に分解する方法を示しています。STLはLOESS(局所的に重み付けされた散布図平滑化)を使用して、3つの成分の滑らかな推定値を抽出します。STL
の主要な入力は次のとおりです。
season
- 季節スムージングの長さ。奇数でなければなりません。trend
- トレンドスムージングの長さ。通常はseason
の約150%。奇数でseason
より大きくする必要があります。low_pass
- ローパス推定ウィンドウの長さ。通常は、データの周期性よりも大きい最小の奇数。
まず、必要なパッケージをインポートし、グラフィックス環境を準備し、データを準備します。
[1]:
import matplotlib.pyplot as plt
import pandas as pd
import seaborn as sns
from pandas.plotting import register_matplotlib_converters
register_matplotlib_converters()
sns.set_style("darkgrid")
[2]:
plt.rc("figure", figsize=(16, 12))
plt.rc("font", size=13)
大気中のCO2¶
Cleveland, Cleveland, McRae, and Terpenning (1990)の例ではCO2データを使用しており、以下にリストされています。この月次データ(1959年1月から1987年12月)は、サンプル全体で明確なトレンドと季節性がみられます。
[3]:
co2 = [
315.58,
316.39,
316.79,
317.82,
318.39,
318.22,
316.68,
315.01,
314.02,
313.55,
315.02,
315.75,
316.52,
317.10,
317.79,
319.22,
320.08,
319.70,
318.27,
315.99,
314.24,
314.05,
315.05,
316.23,
316.92,
317.76,
318.54,
319.49,
320.64,
319.85,
318.70,
316.96,
315.17,
315.47,
316.19,
317.17,
318.12,
318.72,
319.79,
320.68,
321.28,
320.89,
319.79,
317.56,
316.46,
315.59,
316.85,
317.87,
318.87,
319.25,
320.13,
321.49,
322.34,
321.62,
319.85,
317.87,
316.36,
316.24,
317.13,
318.46,
319.57,
320.23,
320.89,
321.54,
322.20,
321.90,
320.42,
318.60,
316.73,
317.15,
317.94,
318.91,
319.73,
320.78,
321.23,
322.49,
322.59,
322.35,
321.61,
319.24,
318.23,
317.76,
319.36,
319.50,
320.35,
321.40,
322.22,
323.45,
323.80,
323.50,
322.16,
320.09,
318.26,
317.66,
319.47,
320.70,
322.06,
322.23,
322.78,
324.10,
324.63,
323.79,
322.34,
320.73,
319.00,
318.99,
320.41,
321.68,
322.30,
322.89,
323.59,
324.65,
325.30,
325.15,
323.88,
321.80,
319.99,
319.86,
320.88,
322.36,
323.59,
324.23,
325.34,
326.33,
327.03,
326.24,
325.39,
323.16,
321.87,
321.31,
322.34,
323.74,
324.61,
325.58,
326.55,
327.81,
327.82,
327.53,
326.29,
324.66,
323.12,
323.09,
324.01,
325.10,
326.12,
326.62,
327.16,
327.94,
329.15,
328.79,
327.53,
325.65,
323.60,
323.78,
325.13,
326.26,
326.93,
327.84,
327.96,
329.93,
330.25,
329.24,
328.13,
326.42,
324.97,
325.29,
326.56,
327.73,
328.73,
329.70,
330.46,
331.70,
332.66,
332.22,
331.02,
329.39,
327.58,
327.27,
328.30,
328.81,
329.44,
330.89,
331.62,
332.85,
333.29,
332.44,
331.35,
329.58,
327.58,
327.55,
328.56,
329.73,
330.45,
330.98,
331.63,
332.88,
333.63,
333.53,
331.90,
330.08,
328.59,
328.31,
329.44,
330.64,
331.62,
332.45,
333.36,
334.46,
334.84,
334.29,
333.04,
330.88,
329.23,
328.83,
330.18,
331.50,
332.80,
333.22,
334.54,
335.82,
336.45,
335.97,
334.65,
332.40,
331.28,
330.73,
332.05,
333.54,
334.65,
335.06,
336.32,
337.39,
337.66,
337.56,
336.24,
334.39,
332.43,
332.22,
333.61,
334.78,
335.88,
336.43,
337.61,
338.53,
339.06,
338.92,
337.39,
335.72,
333.64,
333.65,
335.07,
336.53,
337.82,
338.19,
339.89,
340.56,
341.22,
340.92,
339.26,
337.27,
335.66,
335.54,
336.71,
337.79,
338.79,
340.06,
340.93,
342.02,
342.65,
341.80,
340.01,
337.94,
336.17,
336.28,
337.76,
339.05,
340.18,
341.04,
342.16,
343.01,
343.64,
342.91,
341.72,
339.52,
337.75,
337.68,
339.14,
340.37,
341.32,
342.45,
343.05,
344.91,
345.77,
345.30,
343.98,
342.41,
339.89,
340.03,
341.19,
342.87,
343.74,
344.55,
345.28,
347.00,
347.37,
346.74,
345.36,
343.19,
340.97,
341.20,
342.76,
343.96,
344.82,
345.82,
347.24,
348.09,
348.66,
347.90,
346.27,
344.21,
342.88,
342.58,
343.99,
345.31,
345.98,
346.72,
347.63,
349.24,
349.83,
349.10,
347.52,
345.43,
344.48,
343.89,
345.29,
346.54,
347.66,
348.07,
349.12,
350.55,
351.34,
350.80,
349.10,
347.54,
346.20,
346.20,
347.44,
348.67,
]
co2 = pd.Series(
co2, index=pd.date_range("1-1-1959", periods=len(co2), freq="M"), name="CO2"
)
co2.describe()
/tmp/ipykernel_3881/1071343913.py:352: FutureWarning: 'M' is deprecated and will be removed in a future version, please use 'ME' instead.
co2, index=pd.date_range("1-1-1959", periods=len(co2), freq="M"), name="CO2"
[3]:
count 348.000000
mean 330.123879
std 10.059747
min 313.550000
25% 321.302500
50% 328.820000
75% 338.002500
max 351.340000
Name: CO2, dtype: float64
分解には、データ系列という1つの入力が必要です。データ系列に頻度がない場合は、period
も指定する必要があります。seasonal
のデフォルト値は7なので、ほとんどのアプリケーションでは変更する必要があります。
[4]:
from statsmodels.tsa.seasonal import STL
stl = STL(co2, seasonal=13)
res = stl.fit()
fig = res.plot()
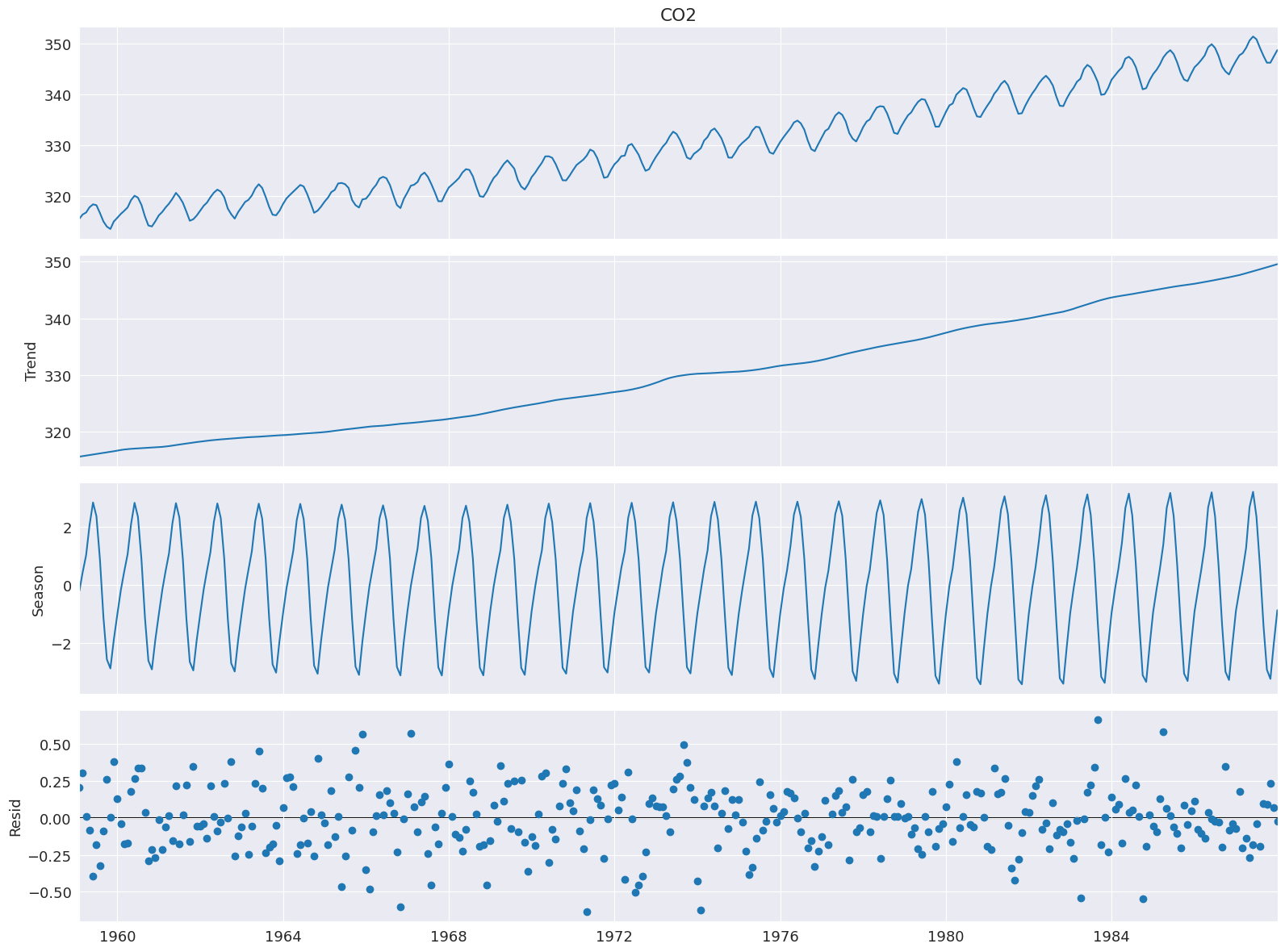
頑健なフィッティング¶
robust
を設定すると、LOESSの推定時にデータを再重み付けするデータ依存の重み付け関数を使用します(LOWESSを使用しています)。頑健な推定を使用すると、下側のプロットに表示されているような大きな誤差をモデルが許容できます。
ここでは、EUにおける電気機器生産量を測定する系列を使用します。
[5]:
from statsmodels.datasets import elec_equip as ds
elec_equip = ds.load().data.iloc[:, 0]
次に、頑健な重み付けありとなしでモデルを推定します。違いはわずかで、2008年の金融危機中に最も顕著です。頑健でない推定では、すべての観測値に等しい重み付けがされ、平均してより小さな誤差が生じます。重みは0から1の間で変化します。
[6]:
def add_stl_plot(fig, res, legend):
"""Add 3 plots from a second STL fit"""
axs = fig.get_axes()
comps = ["trend", "seasonal", "resid"]
for ax, comp in zip(axs[1:], comps):
series = getattr(res, comp)
if comp == "resid":
ax.plot(series, marker="o", linestyle="none")
else:
ax.plot(series)
if comp == "trend":
ax.legend(legend, frameon=False)
stl = STL(elec_equip, period=12, robust=True)
res_robust = stl.fit()
fig = res_robust.plot()
res_non_robust = STL(elec_equip, period=12, robust=False).fit()
add_stl_plot(fig, res_non_robust, ["Robust", "Non-robust"])
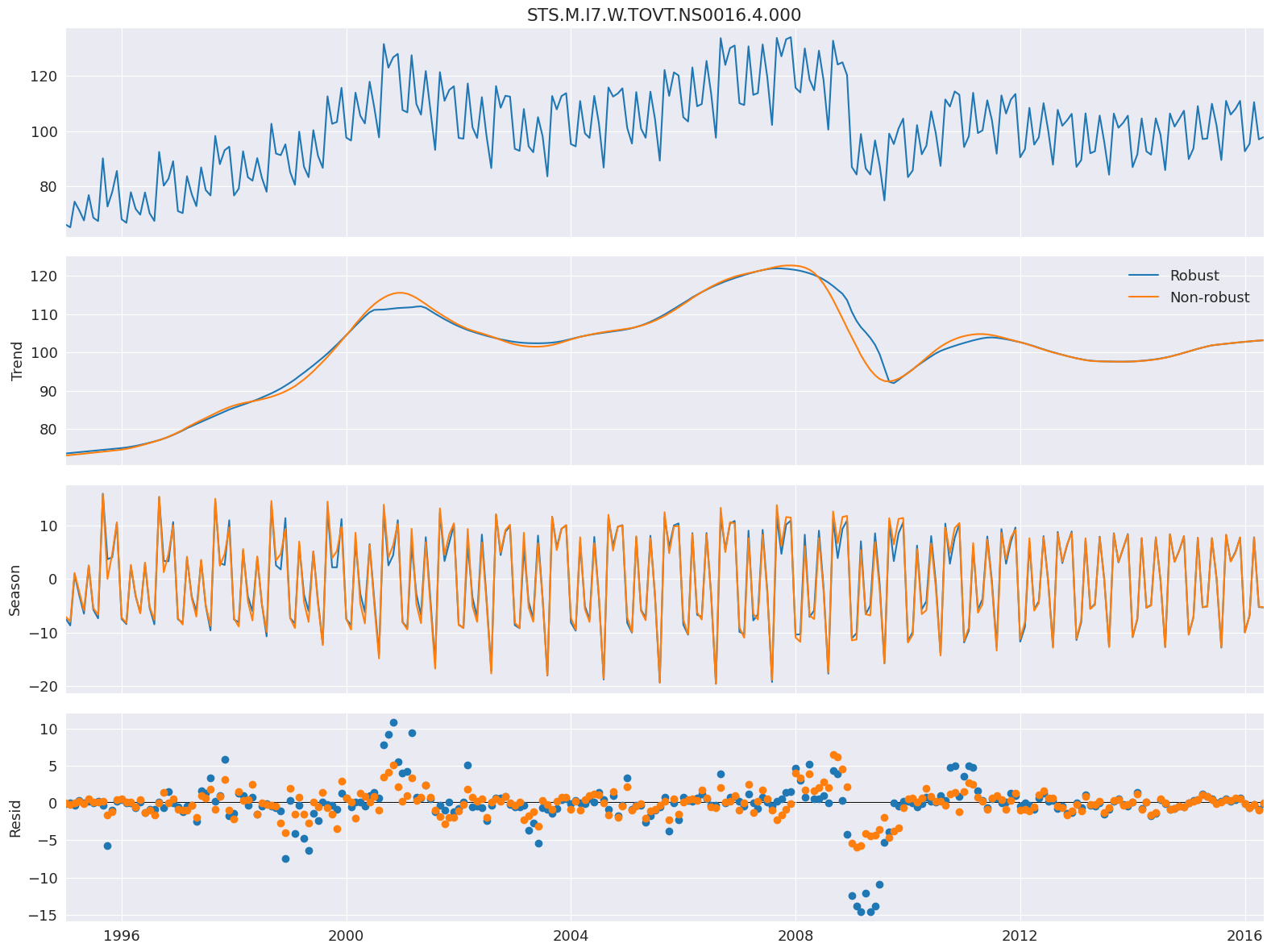
[7]:
fig = plt.figure(figsize=(16, 5))
lines = plt.plot(res_robust.weights, marker="o", linestyle="none")
ax = plt.gca()
xlim = ax.set_xlim(elec_equip.index[0], elec_equip.index[-1])
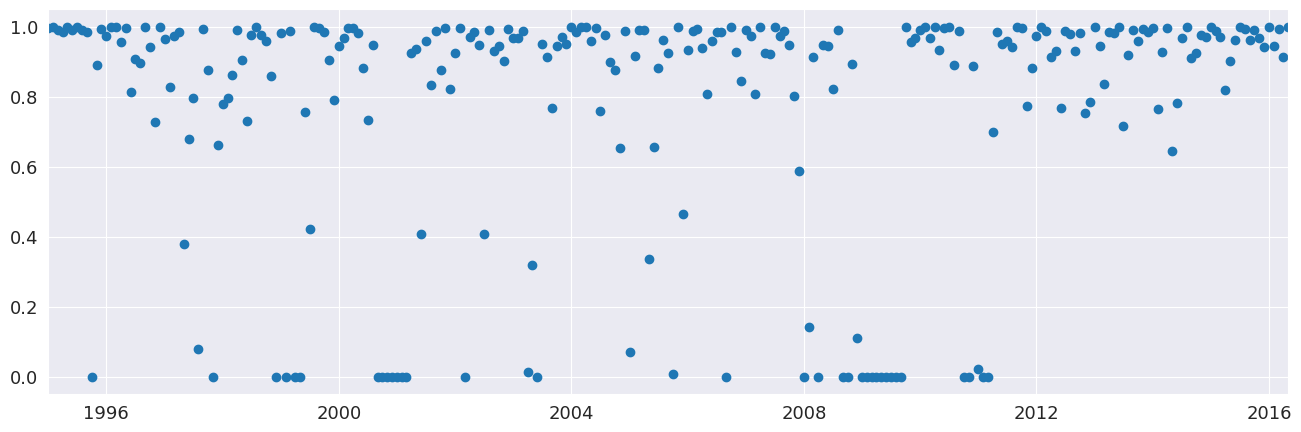
LOESSの次数¶
デフォルトの設定では、定数とトレンドの両方を使用してLOESSモデルを推定します。COMPONENT_deg
を0に設定することで、定数のみを含めるように変更できます。ここでは、2008年の金融危機周辺のトレンドを除けば、次数はほとんど影響しません。
[8]:
stl = STL(
elec_equip, period=12, seasonal_deg=0, trend_deg=0, low_pass_deg=0, robust=True
)
res_deg_0 = stl.fit()
fig = res_robust.plot()
add_stl_plot(fig, res_deg_0, ["Degree 1", "Degree 0"])
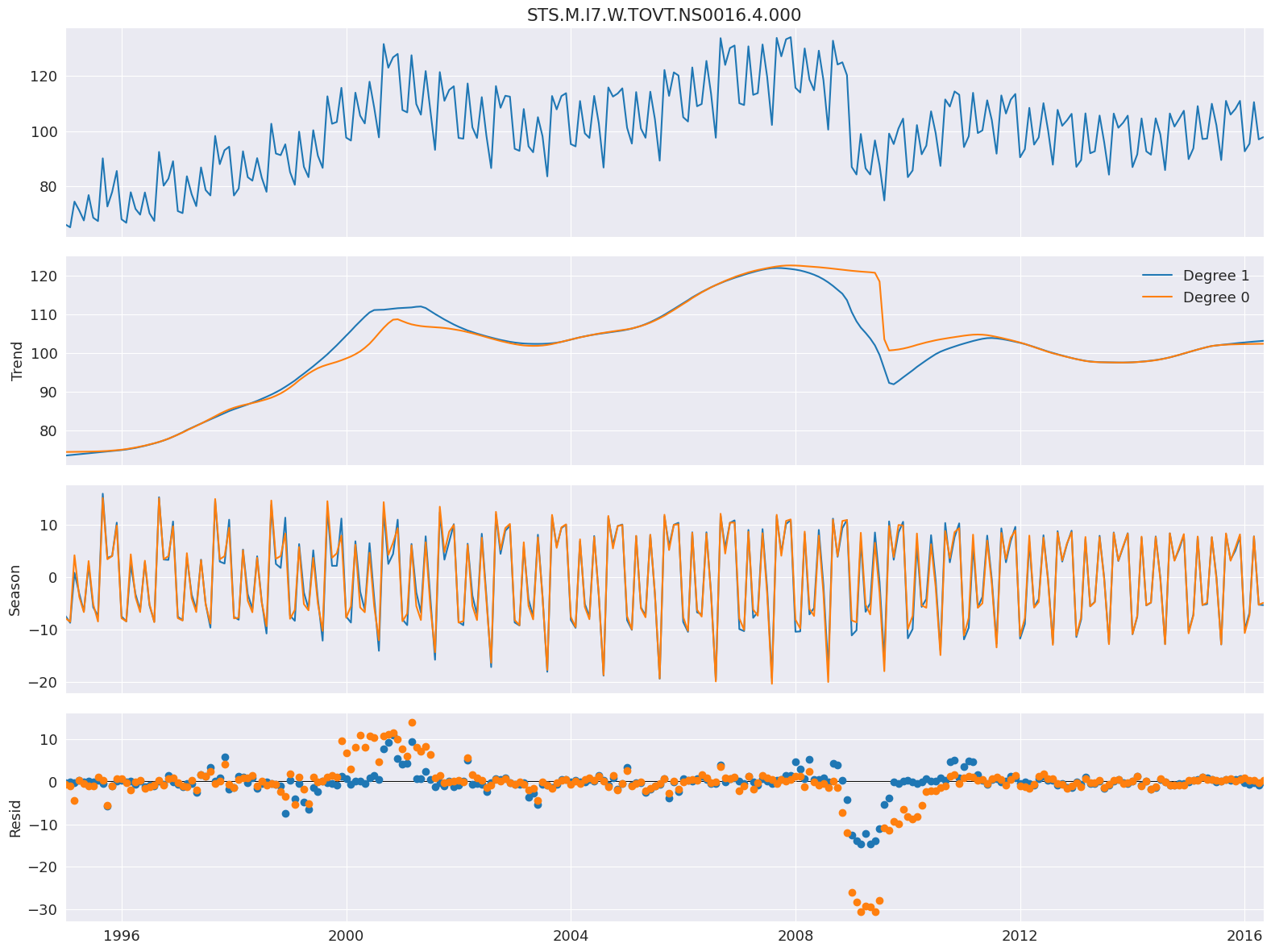
パフォーマンス¶
STL分解の計算コストを削減するために、3つのオプションを使用できます。
seasonal_jump
trend_jump
low_pass_jump
これらの値が0以外の場合、コンポーネントCOMPONENT
のLOESSはCOMPONENT_jump
個の観測値ごとにのみ推定され、線形補間が点間で使用されます。これらの値は、通常、seasonal
、trend
、low_pass
のサイズのおよそ10~20%を超えるべきではありません。
以下の例は、低周波の余弦トレンドと正弦波の季節パターンを持つシミュレートされたデータを使用して、これらが計算コストを15分の1に削減する方法を示しています。
[9]:
import numpy as np
rs = np.random.RandomState(0xA4FD94BC)
tau = 2000
t = np.arange(tau)
period = int(0.05 * tau)
seasonal = period + ((period % 2) == 0) # Ensure odd
e = 0.25 * rs.standard_normal(tau)
y = np.cos(t / tau * 2 * np.pi) + 0.25 * np.sin(t / period * 2 * np.pi) + e
plt.plot(y)
plt.title("Simulated Data")
xlim = plt.gca().set_xlim(0, tau)
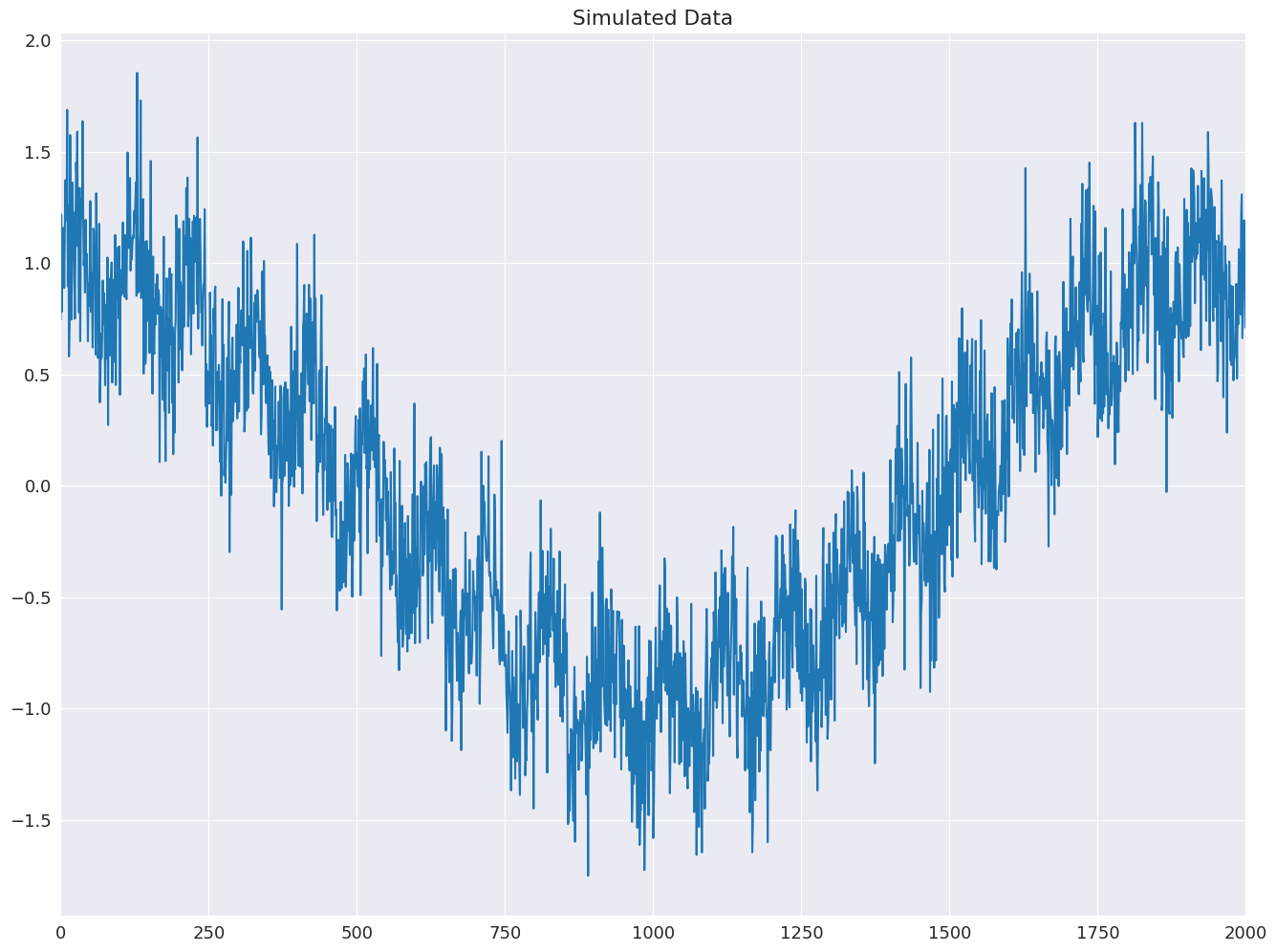
まず、すべてのジャンプが1に等しいベースラインモデルを推定します。
[10]:
mod = STL(y, period=period, seasonal=seasonal)
%timeit mod.fit()
res = mod.fit()
fig = res.plot(observed=False, resid=False)
284 ms ± 38.9 ms per loop (mean ± std. dev. of 7 runs, 1 loop each)
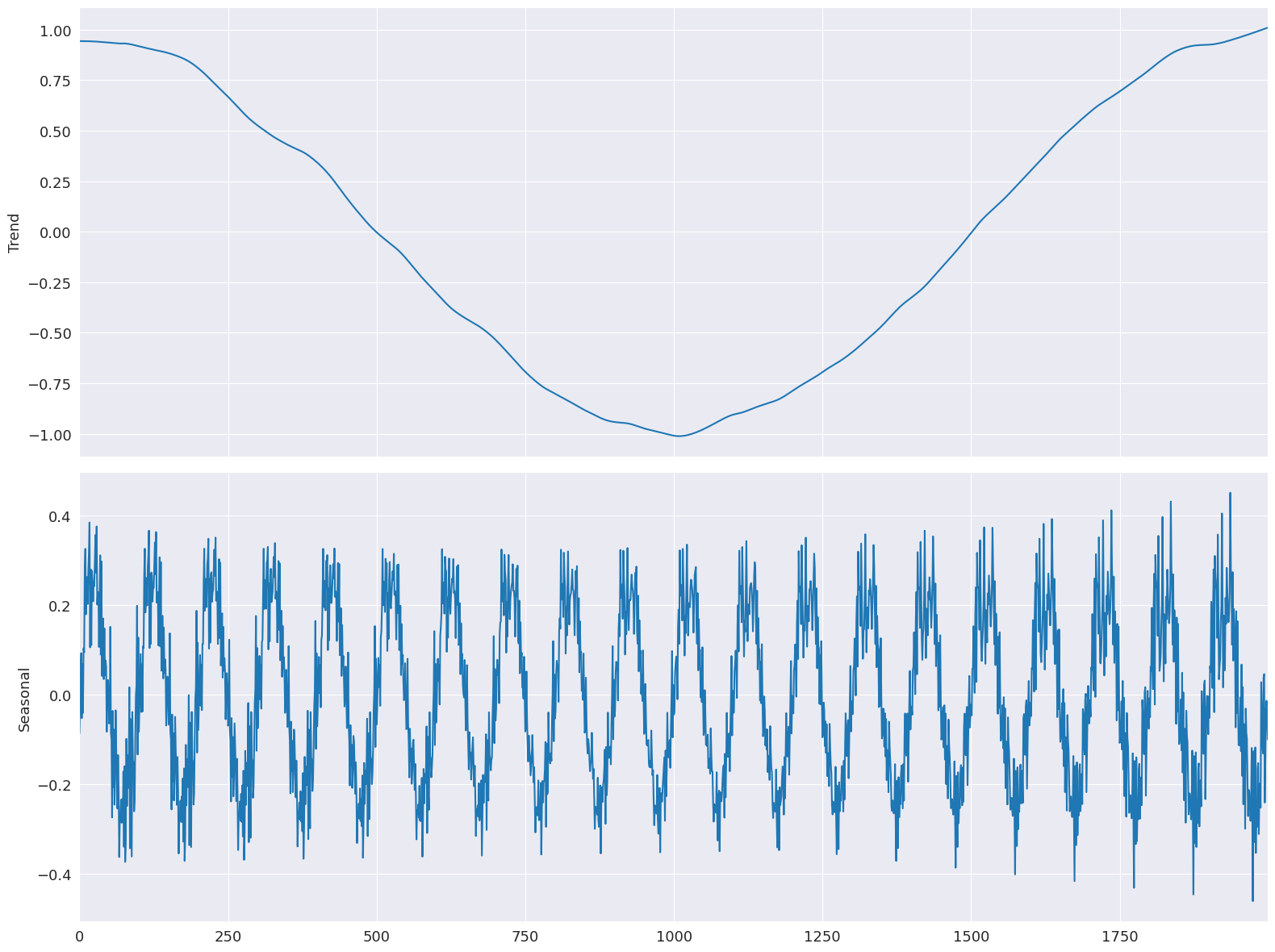
ジャンプはすべてウィンドウ長の15%に設定されます。制限された線形補間は、モデルの適合性にほとんど影響を与えません。
[11]:
low_pass_jump = seasonal_jump = int(0.15 * (period + 1))
trend_jump = int(0.15 * 1.5 * (period + 1))
mod = STL(
y,
period=period,
seasonal=seasonal,
seasonal_jump=seasonal_jump,
trend_jump=trend_jump,
low_pass_jump=low_pass_jump,
)
%timeit mod.fit()
res = mod.fit()
fig = res.plot(observed=False, resid=False)
22.9 ms ± 1.04 ms per loop (mean ± std. dev. of 7 runs, 10 loops each)
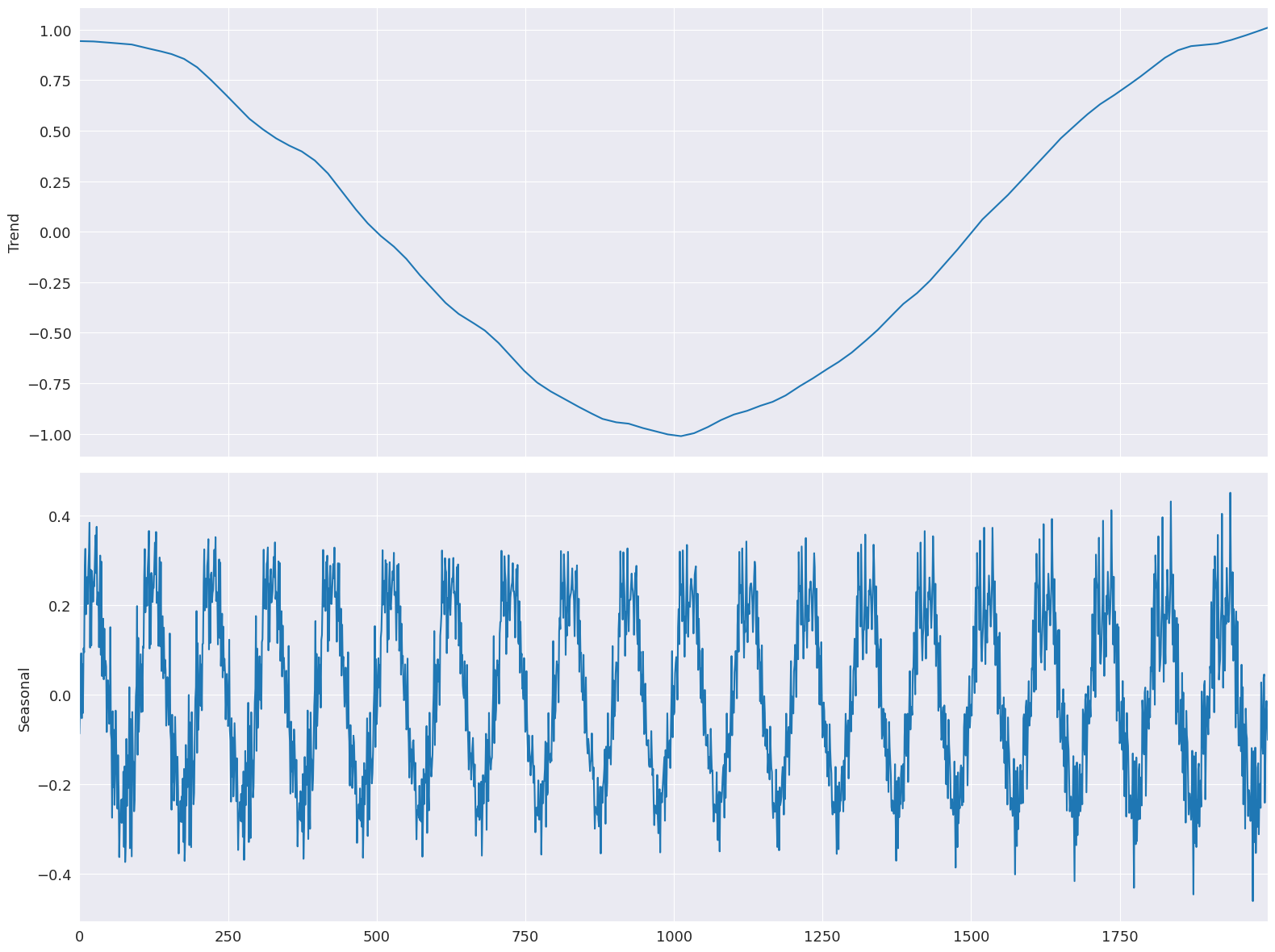
STLを用いた予測¶
STLForecast
は、STLを使用して季節性を除去し、標準的な時系列モデルを使用してトレンドと循環成分を予測するプロセスを簡素化します。
ここでは、STLを使用して季節性を処理し、次にARIMA(1,1,0)を使用して季節調整済みのデータをモデル化します。季節成分は、以下の完全なサイクルを見つけることから予測されます。
ここで、\(k= m - h + m \lfloor \frac{h-1}{m} \rfloor\)です。予測では、ARIMA予測に季節成分予測が自動的に追加されます。
[12]:
from statsmodels.tsa.arima.model import ARIMA
from statsmodels.tsa.forecasting.stl import STLForecast
elec_equip.index.freq = elec_equip.index.inferred_freq
stlf = STLForecast(elec_equip, ARIMA, model_kwargs=dict(order=(1, 1, 0), trend="t"))
stlf_res = stlf.fit()
forecast = stlf_res.forecast(24)
plt.plot(elec_equip)
plt.plot(forecast)
plt.show()
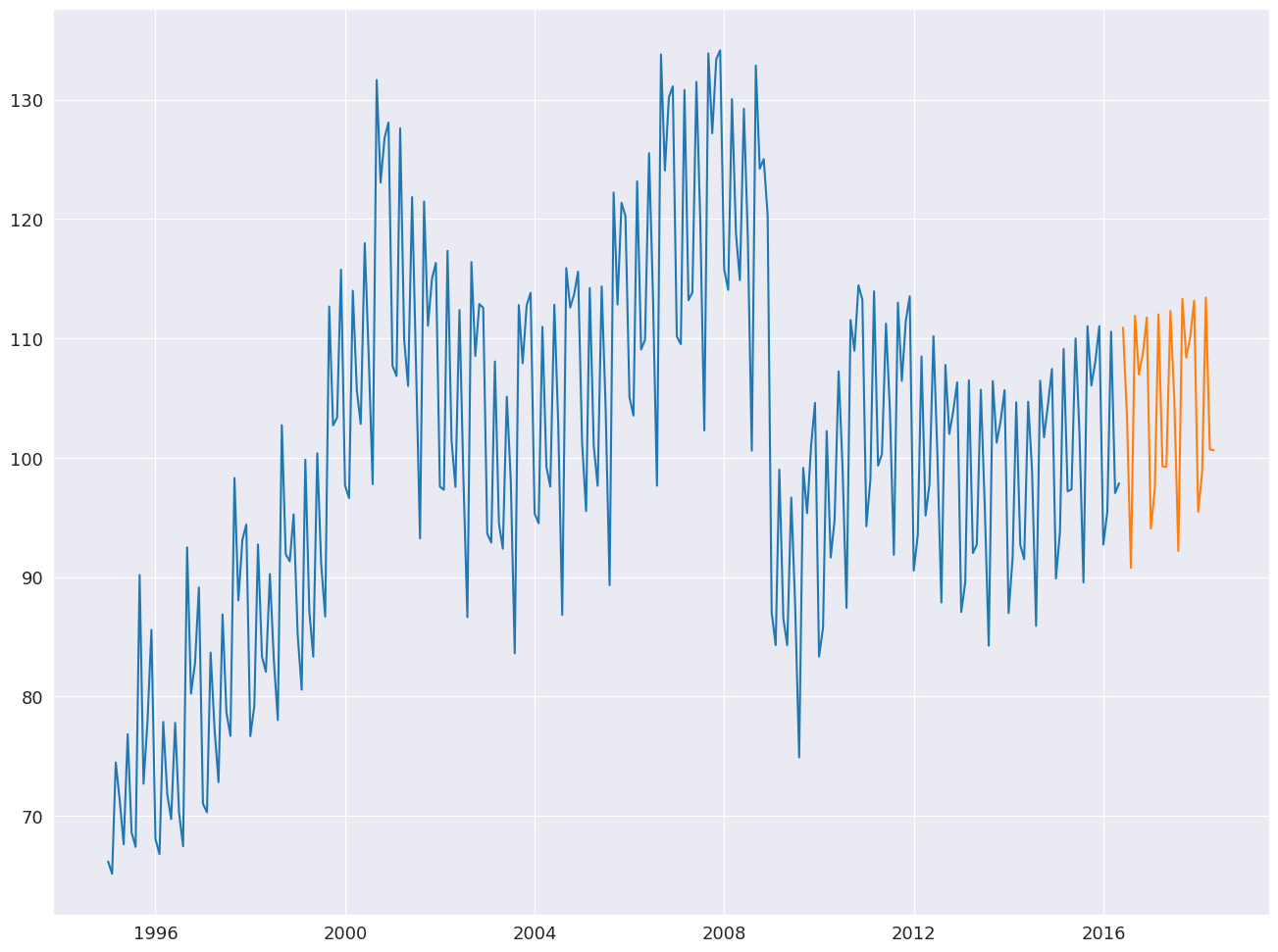
summary
には、時系列モデルとSTL分解の両方の情報が含まれています。
[13]:
print(stlf_res.summary())
STL Decomposition and SARIMAX Results
==============================================================================
Dep. Variable: y No. Observations: 257
Model: ARIMA(1, 1, 0) Log Likelihood -522.434
Date: Thu, 03 Oct 2024 AIC 1050.868
Time: 15:45:57 BIC 1061.504
Sample: 01-01-1995 HQIC 1055.146
- 05-01-2016
Covariance Type: opg
==============================================================================
coef std err z P>|z| [0.025 0.975]
------------------------------------------------------------------------------
x1 0.1171 0.118 0.995 0.320 -0.113 0.348
ar.L1 -0.0435 0.049 -0.880 0.379 -0.140 0.053
sigma2 3.4682 0.188 18.406 0.000 3.099 3.837
===================================================================================
Ljung-Box (L1) (Q): 0.01 Jarque-Bera (JB): 223.01
Prob(Q): 0.92 Prob(JB): 0.00
Heteroskedasticity (H): 0.33 Skew: -0.26
Prob(H) (two-sided): 0.00 Kurtosis: 7.54
STL Configuration
=================================================================================
Period: 12 Trend Length: 23
Seasonal: 7 Trend deg: 1
Seasonal deg: 1 Trend jump: 1
Seasonal jump: 1 Low pass: 13
Robust: False Low pass deg: 1
---------------------------------------------------------------------------------
Warnings:
[1] Covariance matrix calculated using the outer product of gradients (complex-step).